High-Performance Engineering
Big Data is messy. We build distributed, AI-enhanced pipelines to transform billions of data points into visual insights in real time. Here are some examples from our blog below.
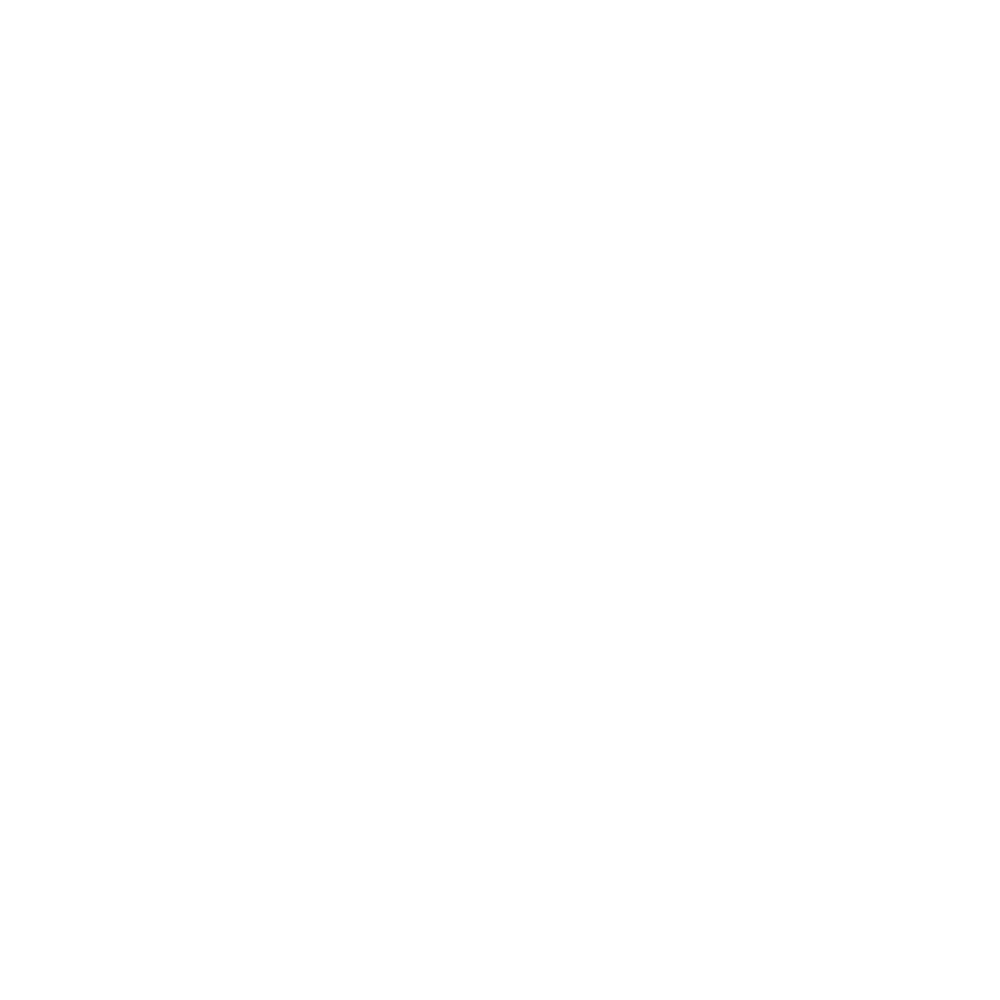
Last summer we began tracking tweets that mention Donald Trump in preparation for our talk at Strata NY. At the time it looked like things were only going to get more interesting so we kept watching and, as of Feb 1, we’d processed over 59 million tweets. With the Iowa caucus last week it seemed like a good time to revisit some of our earlier analysis.
The Tor project is an open network for anonymous communication over the internet. Tor routes users’ internet traffic through a series of volunteer-run relay nodes to conceal its origin and destination from potential surveillance or censorship. While Tor is built for anonymity, the structure of the network and locations of many of the relay nodes is open.
One of the questions we’ve spent a lot of time pondering over the years is a deceptively simple one - How do we visualize billions of data points? To understand why this is a difficult question to answer, let’s look at two popular approaches.
We’ve been writing libraries for Spark at Uncharted for several years, and continuous integration involving the Spark runtime has always been a difficult thing to accomplish. Common approaches, such as creating a Spark context within a standard Scala runtime, can fail to accurately emulate nuances of the distributed Spark environment. I’d like to share a solution we’ve developed for creating a native Spark test environment within TravisCI.